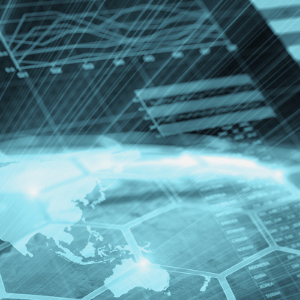
Since the popularization of ChatGPT in December, the availability and adoption of deployable AI models has increased dramatically. While the value derived from such algorithms most certainly extends to financial modelling in the form of improved speed, accuracy, and insights, it is important to understand their limitations and potential biases.
While AI applications in finance include automated data entry or creating automated reports, perhaps the biggest value-add to an end-user of a financial model is the additional certainty that it can provide. While financial analysis skills will most likely always be necessary to make financing/investing decisions, models are now being deployed to help decide on model input assumptions and even evaluate those assumptions. For example, a model could provide a rating between “too optimistic” and “too pessimistic,” such that a potential investor could be provided assurance of the reasonability of the returns, based on the historical performance of models with similar assumptions.1 This may give a cautious investor the conviction they need to make an investment decision.
However, the biggest limitation of AI’s adoption in the financial modelling world is that there are very few(if any)high-performing AI tools built for Excel that can replicate a human’s performance. Part of the problem is that you can’t build an automation tool for something highly susceptible to manipulation and interpretation. For example, analyzing the quality of earnings across two companies requires many judgement calls, making it difficult to quantify and there is significant work to be done until an AI can understand these intricacies wholly.2
While we have seen AI research make leaps and bounds in recent years and even months, they have also become more complex and less understandable. Research has shown that although accuracy is important, medical doctors and business executives alike have rejected their adoption expressing concerns that“AI’s inner workings are too opaque.” 3 In a study where respondents were asked to decide between an interpretable and “black box” model for several use cases such as hurricane and cancer detection, respondents consistently chose interpretability over accuracy. In fact, 74.4% of the decision to use one model over another was reported to be made based on the interpretability of the models.3 Therefore, until public confidence in these AI tools’ ability to produce consistent, accurate, and understandable insights improves, adoption will be slow.
Figure 1: Interpretable vs. Black Box AI/ML Models
While existing AI models provide excellent generalist advice, they tend to perform quite poorly when lacking the correct context for a unique situation, which is almost always the case with financial modelling. However, as these models are continually fed more information to learn from, the insights they can derive will improve and so will their ability to assist in the modelling itself.